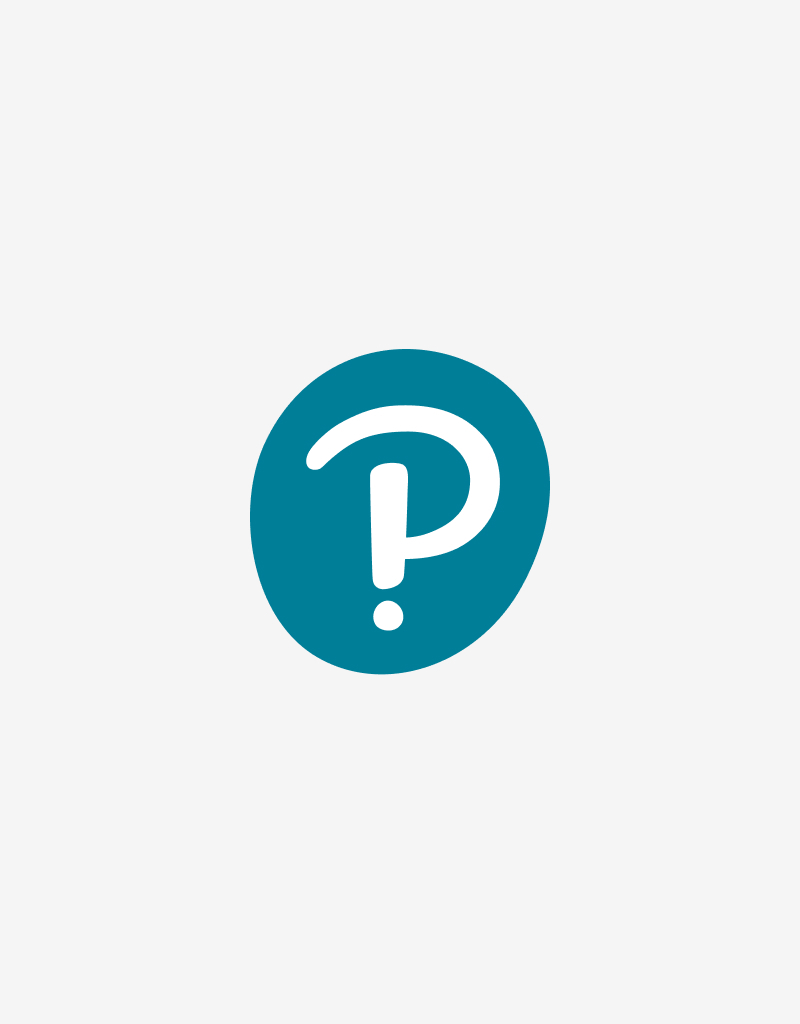
Learning Deep Learning
Magnus EkmanPublished by Pearson (March 29, 2024)
ISBN-13: 9780138177553
Product Information
A complete guide to deep learning for artificial intelligence.
Deep learning (DL) is a key component of today's exciting advances in machine learning and artificial intelligence. Illuminating both the core concepts and the hands-on programming techniques needed to succeed, this video course is ideal for developers, data scientists, analysts, and others—including those with no prior machine learning or statistics experience.
After introducing the essential building blocks of deep neural networks, such as artificial neurons and fully connected, convolutional, and recurrent layers, Magnus Ekman shows how to use them to build advanced architectures, including the Transformer. He describes how these concepts are used to build modern networks for computer vision and natural language processing (NLP), including large language models and multimodal networks.
Introduction
Lesson 1: Deep Learning Introduction
1.1 Deep Learning and Its History
1.2 Prerequisites
Lesson 2: Neural Network Fundamentals I
2.1 The Perceptron and Its Learning Algorithm
2.2 Programming Example: Perceptron
2.3 Understanding the Bias Term
2.4 Matrix and Vector Notation for Neural Networks
2.5 Perceptron Limitations
2.6 Solving Learning Problem with Gradient Descent
2.7 Computing Gradient with the Chain Rule
2.8 The Backpropagation Algorithm
2.9 Programming Example: Learning the XOR Function
2.10 What Activation Function to Use
2.11 Lesson 2 Summary
Lesson 3: Neural Network Fundamentals II
3.1 Datasets and Generalization
3.2 Multiclass Classification
3.3 Programming Example: Digit Classification with Python
3.4 DL Frameworks
3.5 Programming Example: Digit Classification with TensorFlow
3.6 Programming Example: Digit Classification with PyTorch
3.7 Avoiding Saturated Neurons and Vanishing Gradients - Part I
3.8 Avoiding Saturated Neurons and Vanishing Gradients - Part II
3.9 Variations on Gradient Descent
3.1 Programming Example: Improved Digit Classification with TensorFlow
3.11 Programming Example: Improved Digit Classification with PyTorch
3.12 Problem Types, Output Units, and Loss Functions
3.13 Regularization Techniques
3.14 Programming Example: Regression Problem with TensorFlow
3.15 Programming Example: Regression Problem with PyTorch
3.16 Lesson 3 Summary
Lesson 4: Convolutional Neural Networks (CNN) and Image Classification
4.1 The CIFAR-10 Dataset
4.2 Convolutional Layer
4.3 Building a Convolutional Neural Network
4.4 Programming Example: Image Classification Using CNN with TensorFlow
4.5 Programming Example: Image Classification Using CNN with PyTorch
4.6 AlexNet
4.7 VGGNet
4.8 GoogLeNet
4.9 ResNet4.1 Programming Example: Using a Pretrained Network with TensorFlow
4.11 Programming Example: Using a Pretrained Network with PyTorch
4.12 Amplifying Your Data
4.13 Efficient CNNs
4.14 Lesson 4 Summary
Lesson 5: Recurrent Neural Networks (RNN) and Time Series Prediction
5.1 Problem Types Involving Sequential Data
5.2 Recurrent Neural Networks
5.3 Programming Example: Forecasting Book Sales with TensorFlow
5.4 Programming Example: Forecasting Book Sales with PyTorch
5.5 Backpropagation Through Time and Keeping Gradients Healthy
5.6 Long Short-Term Memory
5.7 Autoregression and Beam Search
5.8 Programming Example: Text Autocompletion with TensorFlow
5.9 Programming Example: Text Autocompletion with PyTorch
5.1 Lesson 5 Summary
Lesson 6: Neural Language Models and Word Embeddings
6.1 Language Models
6.2 Word Embeddings
6.3 Programming Example: Language Model and Word Embeddings with TensorFlow
6.4 Programming Example: Language Model and Word Embeddings with PyTorch
6.5 Word2vec
6.6 Programming Example: Using Pretrained GloVe Embeddings
6.7 Handling Out-of-Vocabulary Words with Wordpieces
6.8 Lesson 6 Summary
Lesson 7: Encoder-Decoder Networks, Attention, Transformers, and Neural Machine Translation
7.1 Encoder–Decoder Network for Neural Machine Translation
7.2 Programming Example: Neural Machine Translation with TensorFlow
7.3 Programming Example: Neural Machine Translation with PyTorch
7.4 Attention
7.5 The Transformer
7.6 Programming Example: Machine Translation Using Transformer with TensorFlow
7.7 Programming Example: Machine Translation Using Transformer with PyTorch
7.8 Lesson 7 Summary
Lesson 8: Large Language Models
8.1 Overview of BERT
8.2 Overview of GPT
8.3 From GPT to GPT4
8.4 Handling Chat History
8.5 Prompt Tuning
8.6 Retrieving Data and Using Tools
8.7 Open Datasets and Models
8.8 Demo: Large Language Model Prompting
8.9 Lesson 8 Summary
Lesson 9: Multimodal Networks and Image Captioning
9.1 Multimodal learning
9.2 Programming Example: Multimodal Classification with TensorFlow
9.3 Programming Example: Multimodal Classification with PyTorch
9.4 Image Captioning with Attention
9.5 Programming Example: Image Captioning with TensorFlow
9.6 Programming Example: Image Captioning with PyTorch
9.7 Multimodal Large Language Models
9.8 Lesson 9 Summary
Lesson 10: Multi-Task Learning and Computer Vision Beyond Classification
10.1 Multitask Learning
10.2 Programming Example: Multitask Learning with TensorFlow
10.3 Programming Example: Multitask Learning with PyTorch
10.4 Object Detection with R-CNN
10.5 Improved Object Detection with Fast and Faster R-CNN
10.6 Segmentation with Deconvolution Network and U-Net
10.7 Instance Segmentation with Mask R-CNN
10.8 Lesson 10 Summary
Lesson 11: Applying Deep Learning
11.1 Ethical AI and Data Ethics
11.2 Process for Tuning a Network
11.3 Further Studies
Summary
Magnus Ekman, Ph.D., is a director of architecture at NVIDIA Corporation. His doctorate is in computer engineering, and he is the inventor of multiple patents. He was first exposed to artificial neural networks in the late nineties in his native country, Sweden. After some dabbling in evolutionary computation, he ended up focusing on computer architecture and relocated to Silicon Valley. He previously worked with processor design and R&D at Sun Microsystems and Samsung Research America. In his current role at NVIDIA, he leads an engineering team working on CPU performance and power efficiency for chips used to run artificial intelligence (AI) applications.
As the deep learning (DL) and AI fields exploded in the past few years, fueled by NVIDIA's GPU technology and CUDA, Dr. Ekman found himself in the middle of a company expanding beyond computer graphics into becoming an AI powerhouse. As a part of that journey, he challenged himself to stay up-to-date with the most recent developments in the field. He partnered with the NVIDIA Deep Learning Institute (DLI) and wrote the book Learning Deep Learning, which was published by Pearson in 2021. He is thrilled to now follow up with this video, which is based on the book but also contains additional and updated content about the most recent development in large language models.