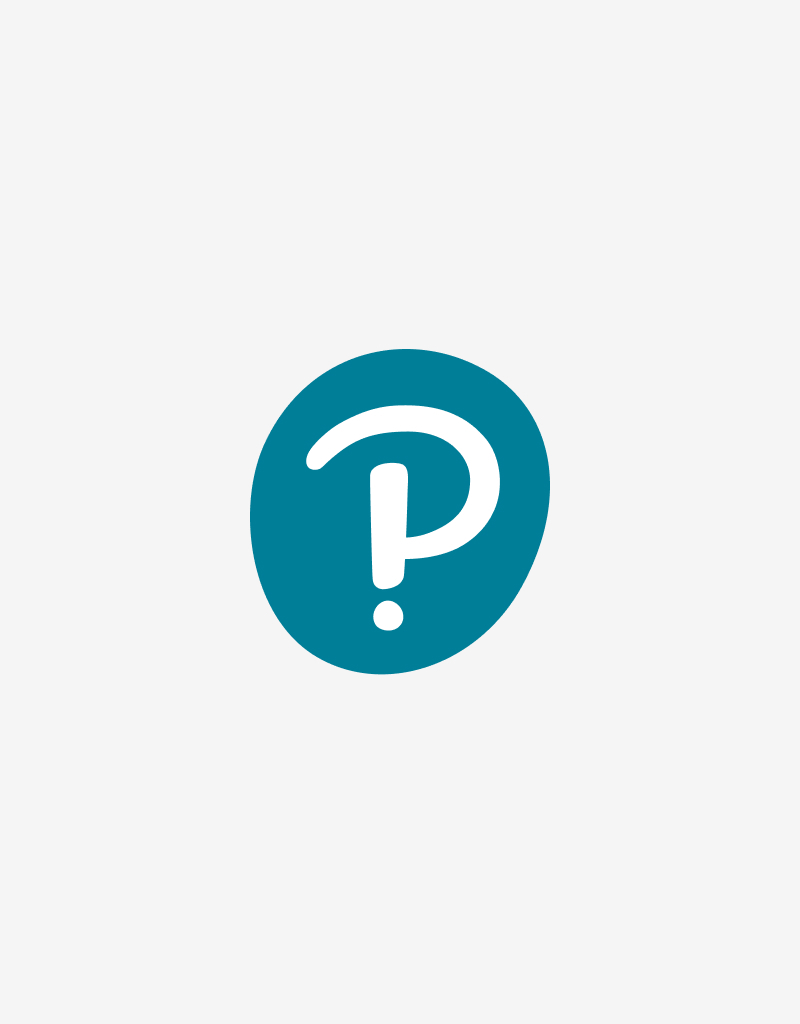
Machine Learning with Python for Everyone, Video Collection
Mark FennerPublished by Pearson (February 10, 2023)
ISBN-13: 9780138092764
Product Information
Machine Learning in Python for Everyone is a collection of courses that teach everything about the foundations and tools for machine learning. As machine learning has moved from futuristic AI projects to data analysis on your desk, you need to begin to build models and start coding machine learning tasks.
Trainer Mark Fenner takes you from introductory machine learning concepts to concrete code. Learn the fundamental metrics used to evaluate general learning systems and specific metrics used in classification and regression. You will also learn about decision tree classifiers and regressors, support vector classifiers and regression, logistic regression, penalized regression, and discriminant analysis.
This collection includes the following courses:
- Machine Learning with Python for Everyone Part 1: Learning Foundations, 2nd Edition
- Machine Learning with Python for Everyone, Part 2: Measuring Models
- Machine Learning with Python for Everyone, Part 3: Fundamental Toolbox
Machine Learning with Python for Everyone, Part 1
Lesson 1: Software Background
Lesson 2: Mathematical Background
Lesson 3: Beginning Classification (Part I)
Lesson 4: Beginning Classification (Part II)
Lesson 5: Beginning Regression (Part I)
Lesson 6: Beginning Regression (Part II)
Machine Learning with Python for Everyone, Part 2
Lesson 1: Evaluating Learning Performance
Lesson 2: Evaluating Classifiers (Part I)
Lesson 3: Evaluating Classifiers (Part II)
Lesson 4: Evaluating Regressors
Machine Learning with Python for Everyone, Part 3
Lesson 1: Fundamental Classification Methods I
Lesson 2: Fundamental Classification Methods II
Lesson 3: Fundamental Regression Methods
Lesson 4: Manual Feature Engineering
Lesson 5: Hyperparameters and Pipeline
Dr. Mark Fenner, owner of Fenner Training and Consulting, LLC, has taught computing and mathematics to diverse adult audiences since 1999, and holds a PhD in computer science. His research has included design, implementation, and performance of machine learning and numerical algorithms; developing learning systems to detect user anomalies; and probabilistic modeling of protein function.